As the consumer markets expand, businesses can struggle with challenges such as streamlining their internal operations, optimizing supply chains, maintaining stock, and managing risks. At the same time, consumers can face roadblocks related to product availability, quality, and the overall purchase experience. Both sets of challenges can be considerably solved when businesses can predict what their target audience will ask for next based on the past data. But sales forecasting is much more than crunching historical data with sales AI tools and generating visualizations.
In this article, let’s understand sales forecasting, look at the advantages it offers, learn about its types and the process itself, and outline the pitfalls you need to watch out for while setting it up for your business.
Contents:
1. Understanding sales forecasting
2. Benefits of sales forecasting
3. Types of sales forecasting methods
4. The sales forecasting process explained
5. Challenges while setting up a sales forecasting process
Understanding sales forecasting
Sales forecasting helps businesses predict how many customers will make purchases and how often they’ll buy in a specific period in the future. This process considers historical sales data, market trends, and customer demand to make an educated prediction.
For instance, let’s say you’re selling cupcakes. If you want to forecast how many cupcakes will be sold in the coming weekend, you can plug in the past weekends’ records into a sales AI tool to make a prediction.
Benefits of sales forecasting
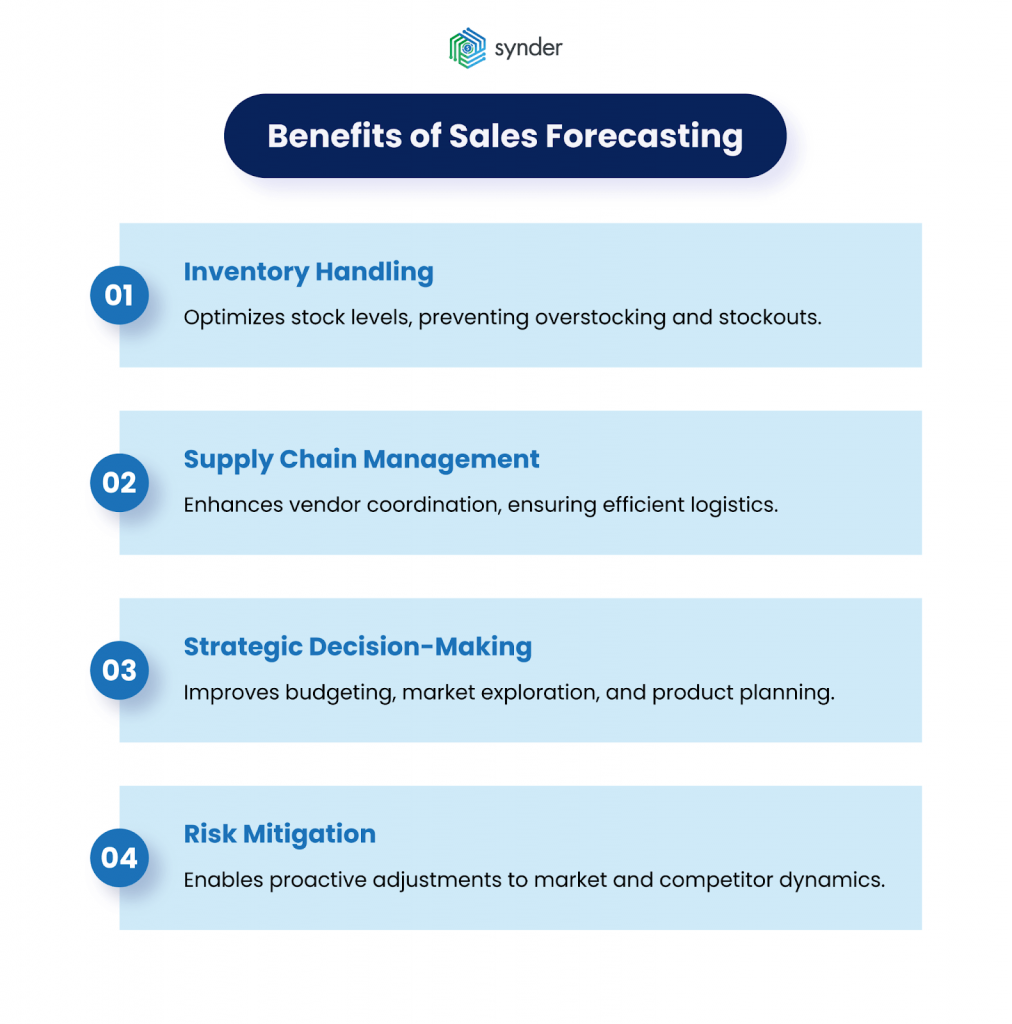
Apart from helping sales teams predict future sales, sales forecasting offers the following benefits:
- Inventory handling: Businesses can easily avoid overstocking and stockouts which will help them serve their customers adequately while decreasing financial loss associated with storage, spoilage, and missed sales.
- Supply chain management: Having estimated sales figures for the upcoming season can be helpful while coordinating with vendors, suppliers, and third-party sales and service providers leading to efficient logistics and quicker response times.
- Strategic decision-making: Sales forecasting can lead to better budget allocation, finding new market opportunities, ensuring the feasibility of expanding business, and planning product launches and promotions.
- Risk mitigation: Forecast data can make businesses agile which is essential for proactively adjusting strategies based on changing factors such as market trends, competitor’s actions, and evolving needs of customers.
Types of sales forecasting methods
Depending on the scope and application, there are three broad types of sales forecasting techniques:
1. Quantitative
The quantitative sales forecasting method relies on historical data, market trends, and customer information and leverages various mathematical models to predict future sales.
As this method utilizes real-world data, you can expect it to give consistent and reliable predictions over time. Additionally, this method can be easily scaled up even when the variety, volume, value, veracity, and velocity increase.
Some popular quantitative sales forecasting techniques include:
- Time series: It considers past sales and market data to predict future sales by assuming that past patterns will likely repeat themselves in the future. This is useful for businesses in stable markets that follow a pattern such as seasonal sales.
- Regression: This method finds how various independent variables like market conditions, price, product features, etc., affect sales based on available data before making predictions. The regression analysis is great for learning the impact of your campaigns and forecasting when multiple factors can affect sales.
- Causal: The causal method goes a step beyond the regression model by mapping cause and effect between your sales figures and the factors that affect them. This can be useful for long-term strategic planning for industries such as healthcare and consumer electronics that are influenced by external factors such as legal regulations.
Various tools within the quantitative sales forecasting workflow implement AI algorithms to improve the accuracy of predictions even when they have limited data making this method a viable option even for small to medium businesses.
You can rely on quantitative sales prediction methods when you have a threshold amount of historical and market trends data available. Furthermore, it also depends on whether a past trend is a good predictor of future performance in your niche or industry.
Due to the nature of the predictions, the quantitative method is usually used to predict medium to long-term sales for a business.
2. Qualitative
The qualitative sales forecasting method relies on the subjective judgment, predictions, and analyses of experts, employees, and external partners. It considers non-tangible information from analysts and other experienced professionals’ reports.
Here’s an overview of the most popular qualitative sales forecasting methods:
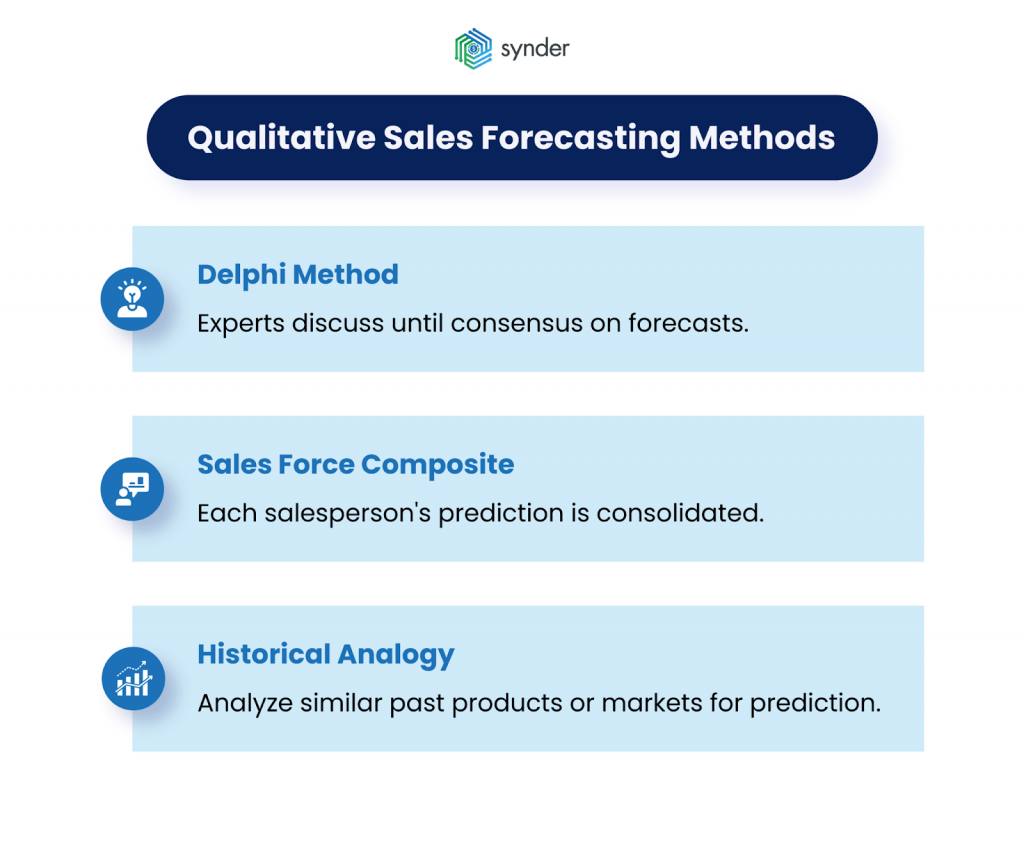
- Delphi method: A panel of experts answer some predefined questions and give their forecasts and opinions. This continues until a consensus is reached. The Delphi method can be useful when a company has multiple stakeholders in their sales department.
- Sales force composite: Here, all of the salespersons provide their individual sales forecasts for an upcoming period and then the predictions are consolidated. This is great for businesses with large sales teams that operate on the field or in retail locations.
- Historical analogy: Experts in a company look at an identical product or market conditions that they’ve gone through in the past to predict the sales forecast. This can be useful for expanding into new geographies with similar audience demographics.
This method is quite affordable and quick as teams don’t have to spend a lot of resources gathering, cleaning, and analyzing data. Moreover, the qualitative sales forecasting technique can be handy when you don’t have enough data or aren’t completely confident in the quantitative analysis.
Entering a new niche or launching a new product, learning consumer sentiment, and managing unpredictable markets are a few conditions when this forecasting method can be particularly useful.
As the qualitative method doesn’t consider past sales data or tangible customer behavior, it’s primarily used for short to medium-term sales forecasts.
3. Term-based
The above two types of sales forecasting methods were based on the nature of information or data considered while making a prediction. The term-based method is based on the time scale of the forecast.
It’s subdivided into three categories:
- Short-term: The time frame can range between a couple of months to a year. This helps businesses with quick operational planning and inventory management.
- Medium-term: This can range between 1-2 years. The insights from a medium-term sales forecast aid businesses in making strategic decisions such as product development.
- Long-term: This usually predicts sales in the 2-year or a longer time scale and informs the decision-makers about the company’s direction, helping with things like investment planning and vision alignment.
The sales forecasting process explained
There are six broad steps in a sales forecasting process. Businesses, based on their requirements, can add or modify the steps to get better results.
1. Defining the objectives of the forecast
The ‘sales’ in your sales forecasting process can predict the purchases resulting from a specific marketing campaign, a product launch, or a new market entry. Determining what sales figure you seek to predict or forecast decides the data points you need to consider, the process you need to follow, and the insights you might gain.
You also need to decide what kind of insights you seek from a forecast. For instance, do you want to improve your inventory management process for the next quarter or make better long-term investments?
2. Select a time frame
You can select an accurate timeframe when you’ve tangibly defined the objectives of the sales forecast.
As we mentioned above, there are three scales of sales prediction: short, medium, and long.
The shorter scales of prediction are better for operational planning and inventory management and tend to be more tangible. The longer scales, although more descriptive and qualitative, are essential for guiding the direction of the business and making long-term growth decisions.
3. Gathering relevant data
This forms the basis on which your sales predictions are made. Sales teams need to ensure their historical data, market trends, economic indicators, and customer information (like preferences and demographics) are reliable.
You can use various tools to collect data from their corresponding sources. For instance, you can rely on a CRM solution like HubSpot to get customer information and Salesforce to gather your past sales data.
4. Generating a reliable forecast
Depending on the available data, resources, and goals of the forecast, choose between qualitative and quantitative methods for an appropriate timescale.
Many analytics tools such as Microsoft Power BI and Tableau can crunch your numbers in a variety of methods to provide you with actionable insights.
To refine the quality of sales forecasts even further, leverage the opinions of experts and experienced members of your organization to complement your analytical findings. This hybrid methodology considers tangible (sales data, market conditions, etc.) and intangible (sentiment, etc.) data while predicting future sales for any timescale.
5. Implementing decisions based on the forecast
After generating predictions, collate them in an understandable format for various stakeholders to determine future action items. During these discussions, it’s crucial to maintain transparency about the methods used and the confidence you have in the sales forecasts as it helps in creating a data-backed action plan.
Businesses have to make decisions related to their inventory, operations, marketing, product development, business strategy, etc., based on the findings of the forecast to optimize the sales revenue.
6. Monitoring and revision
Keep in mind that sales forecasts are predictions and actual business performance may vary, making it important for teams to continuously track these numbers.
For instance, in some cases, certain qualitative methods forecast sales by crunching real-time data which can guide decision-making in business operations.
Additionally, after each quarter or so, brands can compare their forecast against their actual sales figures to tweak their prediction methodology for improved accuracy. This can involve adopting tools that are more suitable for your business, considering new data points, etc.
Challenges while setting up a sales forecasting process
Right now you can speculate that running the sales forecasting process entails understanding various concepts, building a strong and scalable workflow, and constantly iterating it with time, which can lead you to some roadblocks.
Let’s look at four such challenges to find the best ways to overcome them.
1. Inaccurate or incomplete data
The sales forecasting process relies heavily on market trends, historical data, customer information, and surveys to predict what your numbers may look like. If the data is incorrect or lacks certain parameters, it can misdirect you into making wrong decisions.
To overcome this, you can conduct regular data and pipeline audits and educate your team members about the importance of collecting and storing accurate information. Additionally, you can set certain best practices such as validating data before entering it into the knowledge base.
2. Changing market conditions
Disruptive and rapidly evolving technologies such as artificial intelligence are making their way into various aspects of all industries ranging from product innovation to fulfillment. This can make the market volatile, which can render your forecasts obsolete.
Although there’s no definitive way to navigate this, you can adopt flexible predictive models, continuously track trends, and forecast sales for different scenarios to remain agile and take proactive steps to mitigate any risks.
3. Misalignment between departments
Incongruently set workflows and pipelines between marketing, sales, finance, and other departments can lead to inconsistencies in data and ultimately inaccurate sales forecasts.
Businesses should integrate their workflows together (both actionably and technically), encourage cross-departmental collaboration, and adopt dynamic CRM and ERP solutions to gain visibility of various data points across the organization from one place.
4. Balancing qualitative and quantitative forecasts
As explained earlier, future sales predictions can lean either way depending on factors like the timescale of prediction, data points considered, and the objective of the forecasts. With hybrid forecasting methods becoming more common, it can be challenging to find the right balance.
To mitigate this, brands can get multiple experts’ opinions, use dynamic and flexible models to generate forecasts, and listen to their sales teams before determining action items to prepare for the upcoming seasons.
Wrapping up
Sales forecasting helps businesses manage their operations efficiently and make better strategic decisions while delivering their customers better products, services, and experiences.
Businesses, based on the resources available, can predict their future sales qualitatively and quantitatively for short, medium, and long timescales.
To run an efficient sales forecasting process, teams need to define the objectives of the forecast, determine a time frame, gather relevant data, generate a reliable forecast, put the insights uncovered into action, and continuously monitor it for further refinement. Given the complexity of the process, it’s essential to ensure data accuracy, adapt to changing conditions, align inter-departmental processes, and find the right balance between qualitative and quantitative forecasting to get reliable sales predictions.